The peak season is a crucial time of year for logistics and transportation players. During this highly intense period, typically in November and December, carriers must handle millions of parcels every day, multiple times a month. However, such a surge in volumes presents efficiency and profitability challenges for parcel carriers’ networks. Indeed, the peak period often proves to be more costly than profitable, mainly due to its difficulty to predict. It becomes crucial for carriers to prepare it in an optimized way to maximize efficiency and profitability.
The objective here is to provide a comprehensive insight into the logistical implications of the peak season and the formidable challenges it presents. We will delve into how we at Kardinal attempt to address these challenges by leveraging the next-generation algorithms we have developed, and we will conclude by showcasing a real-life case study illustrating our results.
The profitability challenges of peak season
Peak season: a huge impact on parcel delivery companies’ operations
For carriers, the number of parcels to handled during the peak season can be huge, for example:
- GeoPost/DPDgroup: over 370 million parcels delivered worldwide during the peak period, out of a total of 1.9 billion parcels in 2021.
- Hermes: +140 million packages delivered out of 630 million in 2020.
- GLS France: +40% of delivery vehicles deployed as back-up.
- La Poste/Colissimo: between 100 and 120 million Colissimo parcels transported over November-December 2021 period.
The peak season in the parcel delivery industry can be seen as both a lucrative opportunity and a potential source of disruption. With a significant surge in parcel volumes, it undoubtedly offers a chance to boost revenue. However, it also poses challenges due to the sheer scale of demand, which often exceeds the network’s capacity. Balancing these dual aspects is a delicate task, requiring innovative strategies and technology to ensure that peak season remains a period of growth rather than network chaos.
A peak season that does not always ensure profitability
Adapting to the challenges of peak season while safeguarding profit margins is a critical concern for parcel delivery companies. The diagram presented here offers a simplified representation of the parcel delivery network’s organizational structure. Last-mile delivery centers play a central role, collecting and delivering parcels, which are then channeled to hubs and further routed to other hubs based on geographic factors. While the network’s expansion can vary, the fundamental process remains the same, with each parcel collected at one last-mile delivery center and delivered from another.
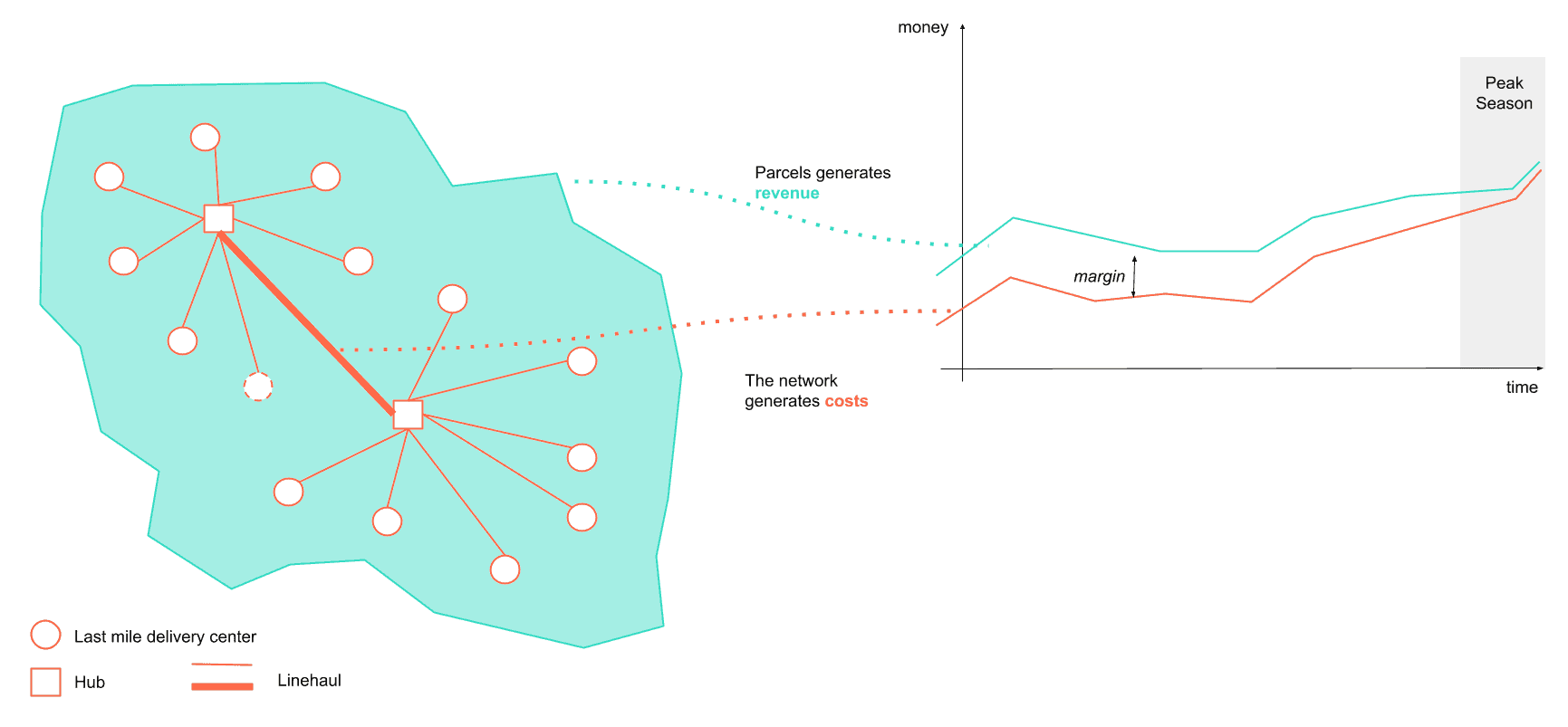
On one hand, carriers generate their revenue from the parcels they deliver. However, parcel volumes exhibit significant volatility, especially during the peak season, and can be challenging to predict. Unforeseeable events such as wars or health crises are likely to completely disrupt forecasts.
On the other hand, carriers’ networks constitute cost-intensive infrastructure that is more difficult to evolve. Building new delivery centers or hubs is a lengthy process, as is altering the organizational practices carried out by operational teams.
Consequently, during peak activity, despite the increase in revenue, costs rise even more rapidly, leading to margin compression, an issue that delivery companies already face on a daily basis.
Adapting to volatile volumes: what strategy?
When faced with the challenge of adapting a network to handle volatile quantities, there are several strategies at your disposal. While we will not cover them all, the fundamental approaches boil down to two options.
First, you can make adjustments to the organization itself. Second, you can adjust the available resources within your network. These options are not mutually exclusive and can be implemented simultaneously. The key lies in the fact that at every level, from the network as a whole down to individual depots and distribution centers, there are various actions you can take. You can modify resource allocation within your network or territory, or you can supplement your resources by adding more.
Combining these actions is how you effectively adapt to fluctuations in volumes. However, this is far from a simple task. Each decision incurs costs, whether in terms of finances or organizational adjustments. It also consumes time, as it’s challenging to implement these decisions efficiently.
This process has a profound impact on the organization itself. Changing the organization often leads to, at least in the short term, decreased performance as individuals need to adapt to new structures and workflows. For instance, when providing a driver or supervisor with a new tool, it takes time for them to become proficient. An effective parcel delivery operation requires a degree of stability, and frequent process changes can create stress and uncertainty.
On the other hand, adjusting available resources, especially during peak seasons, typically involves adding more resources, which inevitably leads to higher costs. Recruiting drivers, who often have limited availability during peak seasons, is a challenging aspect of this strategy. They may only be available for a short period, limiting their efficiency within a given area. As such, minimizing the addition of new resources is crucial, as the impact of such changes can be substantial. Anticipation and careful planning are essential to minimize disruptions, as frequent changes can increase the probability of negative impacts on the network.
Peak season: costs rising faster than revenue
During peak seasons, costs tend to outpace revenue growth, primarily due to the nature of the previously mentioned adaptations. To control these costs, it is essential to make gradual changes. However, achieving this balance becomes challenging when parcel quantity variations are anything but small or smooth.
Often, it is uncertain to predict precisely what the scenario for the next peak season will be, as evidenced by last year’s surprising decrease in volumes.
Therefore, making the right decisions becomes challenging. In most cases, companies find themselves with either undersized fleets, leading to service quality issues, or oversized resources, resulting in unnecessary costs.
Given that maintaining service quality is a top priority, companies tend to err on the side of caution, allocating ample resources to ensure all parcels are delivered. This period is crucial for parcel delivery providers to showcase their ability to handle such surges, particularly for their online retailer clients.
Suboptimal pricing of subcontractors during the peak period
To manage the complex last-mile, parcel delivery companies, especially private ones, heavily rely on subcontractors. This economic model often relies on pricing based on a per-parcel rate. So, when parcel volumes double during peak seasons, the cost paid to subcontractors also doubles linearly. However, does the cost structure of subcontractors also double?
In reality, the cost structure of subcontractors is far from linear. It exhibits jumps and plateaus, as demonstrated in the graphical representation. Each blue dot signifies an actual day of delivery carried out by a subcontractor in a specific area, while the red line represents the price paid relative to the number of parcels.
This analysis reveals that as parcel volumes increase, it becomes more profitable for subcontractors than for parcel delivery companies. This disparity arises because the price negotiated with subcontractors is generally based on nomimal periods and is not sufficiently tailored to the unique demands of the peak season.
Data-driven peak management
Now that we’ve outlined the challenges, let’s explore potential solutions. At Kardinal, we’ve developed algorithms that address real pain points within the last-mile delivery industry. Our “Territory Analytics & Optimization (TAO)” solution is particularly valuable in the parcel delivery sector because it leverages historical data to forecast future scenarios.
A new data-driven paradigm to better predict and prescript the workload plan
To address the challenges posed by the peak season, Kardinal adopts a six-step approach:
- Data gathering: This includes historical data from the previous year’s peak season, with a particular focus on understanding parcel delivery patterns. Additionally, any pertinent internal forecasts, whether derived from client inputs or economic projections, are gathered to provide comprehensive data for analysis.
- Identification of plausibles scenarios for the upcoming peak season: These scenarios are developed at various levels, starting from a global network perspective and then delving into localized considerations, such as delivery centers. Further granularity extends to individual subcontractors, clusters, or teams, reflecting the intricacies of the optimization process.
- Extensive dataset generation: This dataset is derived by extrapolating insights from historical data and intertwining them with the scenarios defined earlier. The objective is to create a comprehensive dataset that accounts for a wide range of potential peak season variations.
- Optimization runs: Leveraging Kardinal’s technology, multiple optimization runs typically amalgamate this data and, for each subcontractor area and cluster, pinpoint the parcel quantity thresholds beyond which a new resource is required to handle the load. These thresholds are established based on a careful analysis of the data, scenario-specific considerations, and historical performance.
- Workload plan: Once the optimization runs are complete, the resulting insights are used to create a detailed workload plan. This plan offers a precise allocation of resources, delineating requirements per area, per week, and per scenario. The intention is to equip either internal teams or subcontractors with a clear and actionable plan for effectively managing resources during the peak season.
- Dashboard visualization: If necessary, the results of the optimization runs are uploaded into the Kardinal platform’s dashboards, which remain available throughout the entire peak season for continuous optimization.
Steps 2, 3, 4, and 5 are explained in more detail below.
Focus on identifying plausible growth scenarios for the future (step 2)
To delve into the predictive aspect further, let’s get concrete with an example from an ongoing study. Here, we aim to identify plausible growth scenarios based on historical data and projections. We consider various hypotheses and create multiple scenarios, ranging from pessimistic to optimistic, depending on the desired level of granularity. Each scenario is carefully planned to cater to different potential outcomes.
Focus on generating data to simulate infinite last-mile complexity (step 3)
The next step involves generating synthetic data—simulated peak seasons that align with past trends and the defined scenarios.
These data are smoothed to avoid overfitting what happened in the past. The forthcoming peak season may deviate slightly from the past, and being prepared for such variations is essential.
The goal is to create a finely detailed projection of data on the territory, providing insights into local knowledge, such as demographics or local consumption patterns and behaviors. For instance, a retailer selling compact lawn mowers may experience fluctuating demand based on promotions, with spikes in deliveries to specific city areas where small gardens are prevalent. Understanding these specificities provides a clearer insight into customer ordering patterns and how they manifest based on geographical areas, helping to better prepare for peak activity periods.
Focus on the resource thresholds identification through massive computations (step 4)
Kardinal’s algorithms play a crucial role in automating the sectorization process, streamlining operations during the peak season.
Take, for example, a specific area where the typical situation involves delivering 400 parcels per day with five drivers. During peak season planning, we identify critical thresholds that trigger the need to add additional drivers. Through the solution, we can identify that as soon as the parcel count reaches 455, an additional driver is required. This configuration remains effective until the parcel count reaches approximately 560, at which point 7 drivers are needed to deliver all parcels.
At each threshold, Kardinal’s solution generates a sectorization plan indicating how to efficiently divide the territory when adding additional drivers. Importantly, this sectorization strategy is designed for resilience, ensuring it remains valid for a range of scenarios within the specified parcel quantity range (in this example: between 455 and 560 parcels).
By following this approach, you can proactively anticipate the required resources and their optimal deployment in the field based on parcel quantities. This not only enhances operational efficiency but also ensures flexibility and resilience in responding to fluctuations during peak seasons.
Focus generating the workload plans (step 5)
Once we have meticulously mapped out the essential thresholds and scenarios, we integrate these elements to create a streamlined solution tailored for operational teams. Our goal is to provide a straightforward tool that equips these teams with actionable guidance, sparing them from intricate diagrams and complex graphs. An example of this tool might appear as follows, with anonymized data:
Depot managers can quickly identify their subcontractors’ activity with the daily planned parcel quantities and delivery routes for each week, enabling them to make informed decisions and adjust resources as needed. For example, they can easily see that in zone 1, an additional driver will be needed in week 45, followed by the need for two more drivers in week 48.
While generating these 2,000 data points and constructing such tables involves extensive computations, Kardinal automates this process, ensuring that operational teams receive clear and actionable insights to optimize their resource allocation effectively.
Case study: optimized peak season preparation with Kardinal’s
Reduction in deployed resources, increase in productivity, and service quality improvement: results of Kardinal optimization
Let’s take a closer look at a practical example to illustrate the impact of our approach. In this anonymized case from a client we assisted last year, the context was particularly interesting. The previous year, they had underestimated their driver needs, resulting in numerous challenges, including delivery rate issues and poor service quality at some distribution centers. Their initial approach was to allocate more resources than necessary to ensure every parcel was delivered on time. However, thanks to the advanced mathematical algorithms at the core of the Kardinal solution, we proposed a more efficient and cost-effective alternative.
The green bars in the graph represent the average parcels per day per week for a specific delivery center. The blue line represents the resources we recommended deploying. Notably, our plan required fewer resources, but it also allowed for a later recruitment phase, giving them more time to adequately train the additional drivers. This had a positive impact on service quality.
The same perspective was reflected in terms of productivity. Their initial plan would have led to a significant drop in productivity, while our approach leveraged activity densification to achieve substantial productivity gains without compromising service quality. This real-world example showcases the effectiveness of our methodology in optimizing resource allocation and enhancing both productivity and service quality during peak seasons.
Global results on delivery rate
The results speak for themselves when we compare the performance from the previous year, denoted as the base year (Y-1), to the year when the Kardinal plan was implemented (Y). As demonstrated in the data, the delivery rate also showed an increase.
This improvement was not only attributed to resource optimization but also to the correction of local inconsistencies and inefficiencies in the tools used for deliveries. The success of our approach was reflected in the satisfaction of subcontractors, who described it as providing a “peace of mind.” They had a clear plan in place, outlining when and how to add additional resources in the field. Following this plan gave them confidence and comfort in managing peak seasons effectively. Overall, the results from the year with the Kardinal plan were significantly more positive and left everyone involved, including subcontractors, much happier compared to the previous peak season.
Here are some key figures that highlight the effectiveness of our approach in this example. The implementation of the Kardinal plan resulted in a nearly 12% reduction in the total number of routes compared to their initial plan. This means they would have planned more resources than we did, and our plan ultimately proved to be closer to reality.
Once historical data was received, it took two weeks to perform 10,000 sectorization optimization runs and provide applicable results for the 67 depot managers and their subcontractors. Such large-scale analysis is not feasible manually, which is why our automated systems excel in handling these calculations and data processing. Our goal is to transform complex mathematical calculations into simple, actionable insights for our clients, streamlining the decision-making process.
In conclusion, managing the peak season in the parcel delivery industry represents a complex challenge that requires innovative data-driven solutions. Kardinal’s approach, based on historical analysis and advanced optimization techniques, provides an effective way to optimize resources and improve service quality while maintaining profitability. This enables delivery companies to better anticipate demand fluctuations and make informed decisions for more efficient peak season management.