Just as in almost every industry over the past decade, Logistics has undergone a profound transformation related to Data. This digitization involves collecting, gathering, and qualifying organizational data to make it usable. Over the years, this process has been associated with buzzwords like Big Data, Data Lake, IoT, Hadoop, Machine Learning, Blockchain, AI. These terms are concepts, technologies, and sciences, often confusing decision-makers rather than clarifying things.
It’s important to distinguish between Data itself and its use. Data, even when stored in accessible, high-performance databases with a high-quality level, is useless on its own: it’s an unexploitable information reservoir for humans, who need tools to use it. What matters is its use, its “activation” by technology. The collection and availability of quality data are only means, not an end. The real ROI of data lies in applications, whose implementation promises to deeply change logistics practices. However, are all applications the same? What promises can they truly fulfill? What links exist between the different levels of data utilization?
Data-Assisted Decision Making
In all contexts, including outside logistics, humans make decisions from the simplest (can I add this package without exceeding my vehicle’s payload?) to the most complex (where should I set up my next logistics hub to reduce costs?). We follow a three-step process:
- Perceive: We gather information about the context.
- Understand: We make sense of this context to describe the problem and anticipate the consequences of our choices.
- Decide: We make the best possible choice with the information we have.
This process is something everyone does daily in all aspects of life.
However, in a context where abundant data is available, its nature (numbers, text, etc.) and quantity (gigabytes/terabytes) make it unusable in its raw state for decision-making. Therefore, humans need tools (or “augmentation”) through technologies to enable this decision-making process effectively.
How? By designing technologies capable of accomplishing these three steps to support and ultimately enable data-assisted decision making. Not all digital era technologies perform these three steps. Instead, there are technologies available to assist at each stage in each of the three levels of data utilization.
Level #1 – Perceiving: Visibility-Oriented Technologies
This first level includes technologies that gather data from multiple sources into a single location. At this stage, it’s about perceiving, giving the user an overview. This involves centralizing data in dedicated storage environments (Big Data) and implementing IoT at different levels of the logistics chain. For instance, installing RFID chips in packages or telematics in vehicles allows knowing their location at any time.
For humans to perceive, collected information must be made available. The main challenge of visibility is information sharing, both within the logistics organization and between organizations. Indeed, the supply chain is often very long, and the links are heavily dependent on each other (a lost package at one level of the chain will “delay” the following levels). Unfortunately, as often, information is siloed between links, meaning each link in the chain experiences the problems of the previous link rather than managing them.
The value of visibility for logistics lies in information sharing, which allows transitioning from a purely reactive mode to problems to a management, process mode. One of its ultimate outcomes in the coming years is the advent of a blockchain for tracing goods transfers between all logistics actors.
However, at certain levels of complexity, raw data alone may not provide enough insight, requiring further processing for valuable conclusions.
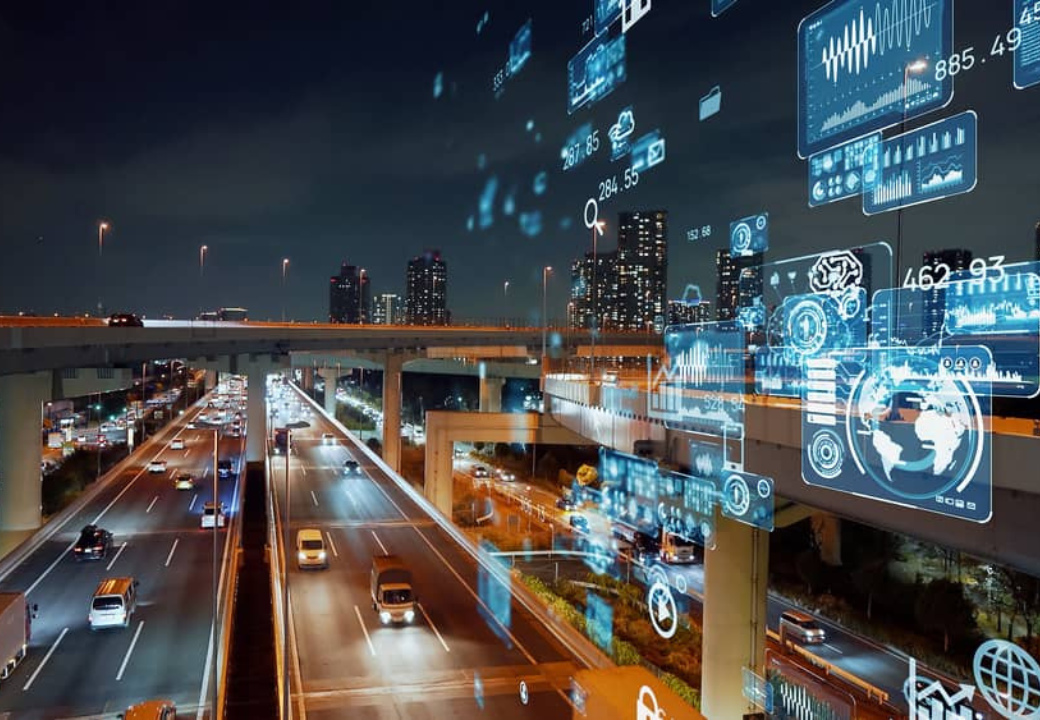
Level #2 – Understanding: Analytical and Predictive Technologies
This second level includes technologies that use statistical data analysis to create other data. Data is “refined” for higher-level use, incorporating Business Intelligence technologies, which often include advanced Machine Learning, to produce reports containing the results of analyzing millions of data lines in a few pages.
These technologies enable discovering problems and studying their potential causes by following more or less complex KPIs. Machine Learning models can, for example, try to explain what causes delivery delays or customer dissatisfaction.
It is also possible to use Data and Machine Learning to anticipate the future and, from history, predict the evolution of KPIs such as demand, raw material prices, etc.
The ambition of these technologies is to provide all the elements, including those that are hard to access, the weak, subtle signals, so that humans can make the best decision.
But there are cases where these tools, while providing interesting insights, do not fundamentally help make the right decision because it is too complex, with too many parameters.
Level #3 – Deciding: Prescriptive Technologies
This last level is the least technologically invested because it also carries the most complex technical and mathematical challenges. It involves designing machines capable of recommending the best possible decision to the user, from all visible and/or predicted data (from levels #1 and #2), among billions of possible decisions.
Humans face these decisions daily, whether optimizing last-mile delivery routes or organizing logistics flows at national and international levels.
The mathematics capable of addressing these issues exist: Operational Research. These are found in end-of-chain technologies, equipping humans making high-impact decisions daily. At these levels of data processing, humans and technology sometimes struggle to find their respective places, sometimes explaining the limited use of these technologies, which are the most capable of helping organizations face future logistical challenges. These technologies still face the challenge of becoming truly operational AI, capable of working well with their users.
Value Creation at Each Stage
Because they leave humans alone with the decision, Level 1 and 2 technologies may sometimes bring less immediate ROI within implementing organizations. Conversely, Level 3 technologies are incapable of producing quality decisions without the groundwork of normalization and qualification from Levels 1 and 2. Just as one cannot properly understand a situation without enough information and cannot solve any problem without understanding it, these three levels are interdependent. They are essential elements in the chain that will allow tomorrow’s logistics organizations to make ever more optimal decisions regarding their costs, customer commitments, and environmental impact.
For logistics actors engaged in digitalization, success lies in equipping themselves to build a complete, coherent, and efficient decision-support chain.